How AI is reshaping marketing and sales
AI is revolutionising organisations’ sales and marketing departments, but managers have generally been slow to exploit this new technology
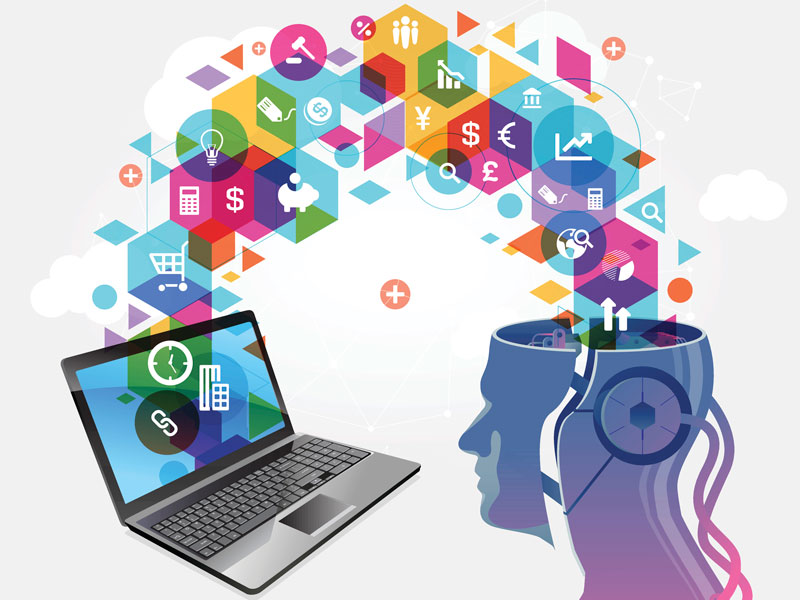
Using AI technology, businesses can process large volumes of data and produce marketing and sales strategies that are tailored to each customer’s purchasing behaviour
The large-scale analysis of data has become customary in most areas of sales and marketing. Now, a new feature of AI, machine learning, is making its mark on corporate operations. With increasingly sophisticated techniques coming into play, marketing and sales teams have the opportunity to deliver massive value if they can translate AI’s potential into concrete change.
AI is the ability of a machine to perform cognitive functions we associate with human minds, such as perceiving, reasoning, learning, interacting with the environment, problem solving and even exercising creativity. Examples of technologies that enable AI to solve business problems are robotics and autonomous vehicles, computer vision, virtual agents and machine learning.
The more data a company has, the more AI applications are possible and the more specialists are needed to develop them
AI is already used in numerous sectors, particularly consumer businesses that have been collecting large volumes of diverse customer data for a considerable time, allowing the development of particularly effective AI systems.
Emerging trends
AI is driving three megatrends in sales and marketing: automation, forecasting and personalisation. These are the areas where marketing leaders should focus their energies to build capabilities
Automation allows machines to make decisions, or at least to assist human decision-makers. Typical examples include dynamic pricing and systems for product recommendations. Automation is currently particularly relevant in online retail and has clear disruptive potential. This will only become more pronounced as technology evolves to make completely independent decisions.
Forecasting depends on having a strong set of historical data to feed AI, which can then find patterns and develop models for future scenarios. In this way, we can predict typical customer behaviour, such as purchase decisions or patterns of fraud. These systems are not static; they are always learning and adapting to new data contexts.
Personalisation refers to the application of forecast results to tailored customer segments and individuals. Using comprehensive customer information, the system analyses each person to make carefully targeted, personalised promotions, products and price offerings.
Real-world application
One application of AI might work as follows: a department store chain wants to boost sales in its cosmetic department and decides to provide more tailored information about the offering to its customers.
Using data gathered from loyalty cards, it develops an AI system to identify purchase patterns for each customer and predict their future needs. For example, a customer might tend to buy a certain perfume if they have selected their shower gel shortly beforehand.
Using this information, the company could send the customer a newsletter featuring products that are tailored to their purchase behaviour. AI systems then learn from customer responses and adjust messages and subsequent offers accordingly.
This results in more relevant offerings for customers and an increase in customer satisfaction, leading to higher sales.
AI is particularly powerful in this context because it is able to execute such a scenario on a large scale, meaning it can deliver personalised marketing to every consumer.
Reaping rewards
To exploit the benefits of AI, organisations should focus on three areas: data strategy, data expertise, and marketing and commercial talent management.
First, it’s important to develop a clear data strategy. It is only possible to develop effective AI systems when we have a sufficient volume of data. As such, key questions must be answered: how can we collect more customer data even faster? How can we safeguard data quality and eliminate bias? How can we achieve this without breaching compliance or data protection regulations?
Second, we must look at the recruitment of AI and data experts. The more data a company has, the more AI applications are possible and the more specialists are needed to develop them. Businesses should hire experts in a range of fields, such as data engineering, data science and software development, to be sure that AI systems function effectively and produce useable results.
Third, we must consider the initial training and ongoing professional development of ‘traditional’ sales and marketing staff. It is up to these employees to convert the results produced by AI systems into added value.
This is often the point at which employees are not motivated to exploit the opportunities offered by AI systems. An important goal should be to train marketing and sales teams so they understand enough about data analysis to translate entrepreneurial problems into AI questions and can then convert the corresponding results into better decisions and actions.
As processing power and data harvesting develop, AI systems will become smarter. But how effective those systems are depends on how well organisations can generate answers and act on them to build commercial value.