The troubling influence algorithms have on how we make decisions
People are quick to accept the credibility of computer-generated search results, but algorithmic tools are as flawed as their makers
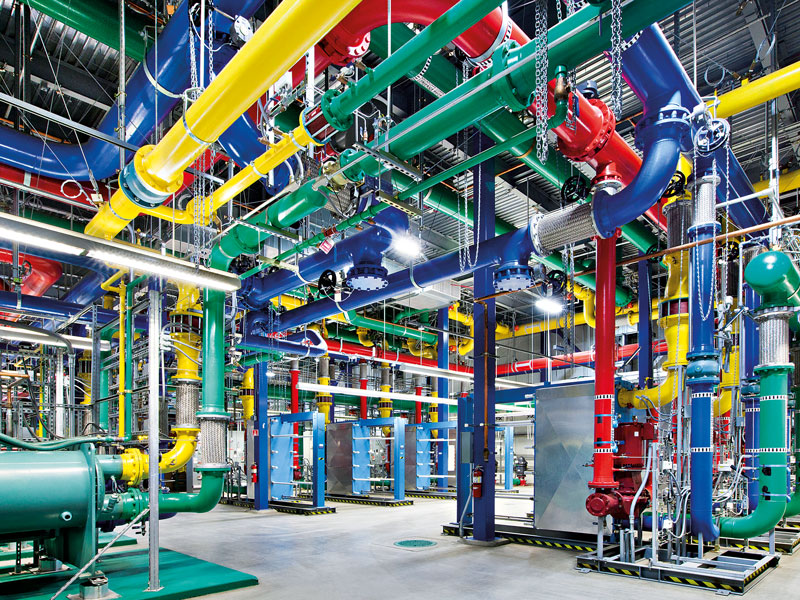
A Google data centre
Google co-founder Sergey Brin once quipped: “The perfect search engine would be the mind of God.” But while Google’s uncanny ability to regularly find exactly what you are looking for may seem divine, in reality, even the most sophisticated uses of machine learning have already run into a wall of human fallibility and immorality.
At their heart, algorithms are simply tools that learn from large swathes of data, providing some sort of optimised response – like a ranking or rating – in accordance with a pre-programmed procedure. They have a reputation for being infallible, neutral and fundamentally fairer than humans, and as a result are quickly making their way into both commercial and public decision-making. While some may still seem far-fetched, we are already far more reliant on algorithmic decision-making than most people know.
The implications of delegating our decisions to these tools range from perpetuating fake news and conspiracy theories to unfairly lengthening the prison sentences of minority groups. Far from being neutral and all-knowing decision tools, complex algorithms are shaped by humans, who are, for all intents and purposes, imperfect. Algorithms function by drawing on past data while also influencing real-life decisions, which makes them prone, by their very nature, to repeating human mistakes and perpetuating them through feedback loops. Often, their implications can be unexpected and unintended.
A moral dilemma
Take Google, for example. Around 3.6 million searches are made through the company’s search engine every minute; I would question anyone who claimed their beliefs had never been shaped to some degree by the information they found through the site. When Google responds to a query, it considers a huge variety of metrics in order to ultimately decide which web pages to include, prioritise and exclude. The date a page was created, the number of keywords it mentions, the ‘reading level’ of the text, the number of inward and outward-bound links, and the ‘authoritativeness’ of the site are just a few of the components that will determine a site’s page ranking. There are hundreds of such criteria.
Like most machine-learning algorithms, Google not only analyses our behaviour: it shapes it
At times, this combination of carefully balanced metrics can cough up highly questionable results. Consider the fact that, for some time, Google’s algorithm responded to the query “did the Holocaust happen?” with a series of pages promoting Holocaust denial – the first of which was a page by a neo-Nazi site entitled ‘top 10 reasons why the Holocaust didn’t happen’. What’s more, autocomplete suggestions have been found to prompt searches for “climate change is a hoax” or “being gay is a sin”, with the top-ranking sites acting to confirm those notions.
Often, the search engine simply acts to perpetuate society’s ready-made biases. Some of the ugliest manifestations of this can be found in the autocomplete feature of the search bar. For instance, typing “why are black women…” has been found to autocomplete with “…so angry” and “…so loud”. Google’s Knowledge Box also falls foul of some grossly offensive untruths. The search term “are black people smart?” for some time responded with: “Blacks are the least intelligent race of all.”
The issue is that some of the seemingly harmless metrics Google employs can learn from the available data in such a way that prioritises harmful results. A key determinant of ranking is the number of page views (or popularity) a link has built up. This can lead to the site favouring extremes of opinion by promoting particularly shocking or ‘clickbait’ items. Some argue this, among other factors, has resulted in a systematic bias towards extremist views.
Furthermore, other metrics such as frequency of appearance, which is used to rank stories on Google’s news page, could lead to political bias if one election candidate receives a greater level of media attention. If popularity correlates with other factors, such as racial divides, this too will cause the results to be biased. The prioritisation of popularity as a metric can also mean the authority or truthfulness of a story can be sidelined in favour of its superficial draw – this has been shown by Google’s Snippets feature, which has been tricked by fake news stories in the past. What’s more, people trying to game the system could hijack any one of Google’s metrics, further exacerbating issues of bias.
The decisions Google’s algorithm makes, therefore, are important, particularly considering the ever-present potential for feedback loops. Like most machine-learning algorithms, Google not only analyses our behaviour: it shapes it. For instance, the popularity of a search term is a factor that drives it higher in the rankings, leading to what psychologist Robert Epstein calls the “digital bandwagon effect”. Epstein explained: “Popularity pushes one viewpoint – or candidate – higher in rankings; higher rankings increase the popularity of that viewpoint. This goes round and round until one viewpoint dominates people’s thinking.”
In April, Google released a blog post acknowledging its role in the proliferation of fake news, hoaxes, unsupported conspiracy theories and offensive content, noting: “[It has] become very apparent that a small set of queries in our daily traffic (around 0.25 percent) have been returning offensive or clearly misleading content, which is not what people are looking for.”
Yet, while these cases may be seen as extremes, issues also exist on a much broader level. For example, if you search “great poet”, the results are predominately white men. If you search “beautiful woman” under images, your result is likely to be a long stream of white women’s faces that match a narrow definition of beauty.
In Google we trust
Google may have little control over the existence of problematic content on the web, but it does control the information its algorithm pays attention to. “These machines will learn what you teach them: the only way that we can get them to be polite is to pass on some human savvy,” noted Jacob Metcalf, a researcher at Data & Society.
At times, it seems likely the algorithm is hand-fed specific commands. For instance, when Googling “FTSE 100 CEOs”, the lead ticker presents the user with five women and five men. This appears to be a conscious effort to counter a stereotype, especially given just seven FTSE 100 CEOs are women, and those companies are by no means the most prominent. Similarly, many of the examples previously mentioned have since been tweaked.
But the company’s ability to make tweaks or adjustments to avoid such results places a lot of power in its hands. These decisions are akin to programming a moral compass into the index of the internet; undoubtedly, this has steep implications. Meanwhile, the secretive nature of the algorithm also means people cannot scrutinise the decisions it makes. For example, when Google faced heavy public pressure for its role in proliferating fake news, it made a characteristically vague announcement that it had taken action by adjusting the “signals” its search tool uses, shifting a greater focus to the “authority” of a page.
The secretive nature of algorithms means people cannot scrutinise the decisions they make
“We have to demand to know what kind of influence these algorithms have over us,” said data scientist Cathy O’Neil, whose work focuses on exposing the implications of algorithmic decision-making. While the secretive nature of Google’s algorithm itself makes it hard to delve into such questions, research has begun to shine some light on the issue.
Most notably, Epstein recently directed a study that aimed to assess the effects of search engine rankings on people’s political beliefs. Participants in his study were presented with real internet pages while using a simulated search engine, which provided different ranking orders for different groups in the study. Unsurprisingly, he found that after being exposed to the search engine, people’s beliefs were strongly affected by the stance of pages located at the top of the rankings, an effect he calls the ‘search engine manipulation effect’ (SEME).
What is particularly striking is the influence of SEME. According to Epstein, SEME is one of the largest behavioural effects ever discovered in the field of psychology: “With a single exposure to search results that favour one candidate or viewpoint over another, we get a very large shift in people’s opinions – up to 80 percent in some demographic groups. This occurs even though people cannot detect the favouritism in the search results; in other words, the manipulation is invisible to people.”
As for the reason behind SEME, Epstein references the famous experiment conducted by psychologist Burrhus Frederic Skinner, in which rats were conditioned to adopt certain behaviours by administering a punishment or reward in response to specific actions. “Like rats in a Skinner Box, our daily experience with a search engine keeps teaching us what is at the top of the list is best,” Epstein said. “That’s why high-ranking search results have such a dramatic effect on people’s opinions, purchases and votes.” Most disturbing, perhaps, is that we are prepared to trust whatever Google offers us despite knowing the information we are reading is likely subjective.
Ruthless robots
Google is an algorithm that we are all familiar with, but it is far from being the only algorithmic decision-making tool influencing our daily lives. “Algorithms are now involved in many consequential decisions in government, including how people are hired or promoted, how social benefits are allocated, how fraud is uncovered and how risk is measured across a number of sectors,” said Nicholas Diakopoulos, Assistant Professor at the Northwestern University School of Communication.
As with Google, it is often only in retrospect that people notice when questionable results start to crop up. For instance, it only recently emerged that Facebook’s algorithm-based advertising feature enabled advertisers to specifically target anti-Semites. What’s more, it was only after some time it emerged LinkedIn’s algorithms suggested male names more frequently than female ones. One of the most disturbing discoveries, however, surrounds the risk scores currently used in criminal courts.
3.5bn+
Searches per day
40,000+
Average number of queries answered per second
0.25%
Estimated number of queries returning offensive or misleading content (as of April)
In the US, an algorithm called COMPAS is often used to predict the likelihood of a defendant reoffending. When someone is booked into jail, they are taken through a questionnaire that covers topics such as previous arrests and childhood upbringing. The software then uses this information to calculate a ‘risk score’, which is provided to judges as guidance for decisions regarding bail and the length of sentence. The ethnicity of the defendant is never revealed, but a recent investigation by non-profit organisation ProPublica indicated the software’s results were systematically disadvantaging black people.
When ProPublica delved into data on risk scores and the criminal histories of 12,000 offenders in Florida, it found the algorithm was more likely to mistakenly brandish a black person as high risk than a white person. A similar bias occurred at the other end of the spectrum, with a greater number of white people being wrongly categorised as low risk. In reality, of those in the low risk category, white people were almost twice as likely to be rearrested than black people. Once again, this troubling bias emerges from the algorithm’s use of past data to make predictions, meaning any correlations picked up in historic datasets will affect decisions made in the future.
Algorithmic accountability
Diakopoulos points to the fact that while the problematic implications of many algorithms have been exposed, we may have only just begun to skim the surface. As such, Diakopoulos is leading a project that hopes to prompt investigations into algorithms currently being used or developed by the US Government: “We have more than 150 algorithms – 159 to be exact – on the list that we’ve identified as being potentially newsworthy if investigated further. These algorithms come from a wide variety of agencies across the federal government including everything from the Centres for Disease Control [and Prevention] and the Department of Veterans Affairs to the National Park Service.”
To narrow in on a single example, the ‘assessment decision tool’ is an algorithm designed to help HR professionals develop assessment strategies for skills and competencies, as well as other situational factors relevant to their hiring criteria. According to those on the project, this algorithm needs to be reviewed in case it is systematically excluding appropriate candidates.
The key issue the project hopes to target is transparency. Many of the most consequential algorithms currently being used in the public and private domains are complex and opaque, making it hard to attribute accountability to their actions. O’Neil also believes accountability is key: “If they restrict our options, and they take away options from us – a job, loan or affordable insurance – then we should have the right to understand it much more than we do now.”
Inside the black box
Improving transparency, however, is no easy task. Companies with algorithmic products would lose their competitive edge if they were forced to make their algorithms public. But, according to Epstein, there is one way to leapfrog this problem: “Transparency is not enough. In fact, because algorithms are quite complicated, information about Google’s algorithms would be useless. This is a simple matter. The index to the internet should be a public instrument, owned and controlled by the public. It should be a public utility. It should be an index, pure and simple – not a tracking device or a mechanism of manipulation. Google doesn’t own the internet, and it also should not own the index to the internet.”
Perhaps all that’s needed is a requirement to certify if an algorithm is safe or fair to use, and greater incentives to pay attention to the consequences of an algorithm once it’s up and running. According to Metcalf: “What we really need are review committees, we need policies within companies, we need codes of ethics, we need to have the cultures, habits and infrastructures to rigorously and efficiently pause and ask the question about who algorithms are affecting, how are they affecting them and how they might be improved. These moments don’t really exist in current engineering practices with any real sense of rigour.”
The most attractive option is to put the control of algorithms back into the hands of the people that are affected by them. In practice, this might work through the creation of a settings feature, where people can set their own personal algorithmic preferences. One would be given the option to instruct Google, say, to pay particular attention to location, popularity or timeliness when ordering results.
The same concept could feasibly be rolled out in all sorts of locations. Metcalf noted one of the most interesting applications of this idea would centre on autonomous vehicles. When life-or-death decisions arise, such as whether to save the driver or the pedestrian, humans make their own decisions in the moment. “With a machine, there has to be some sort of instruction,” Metcalf said.
“There is a suggestion that rather than letting the manufacturers decide, we should let the drivers decide. When I go and buy my new autonomous Mercedes, there should be a slider bar when I log in for the first time saying ‘do I save your life or do I save the pedestrian’s life?’” Such decisions are human by their very nature, and should be treated as such. Who knows, an algorithmic slider could, one day, form part of our daily lexicon. But, in the meantime, algorithms need to be managed; ensuring those with the power to shape our lives do so with some code of conduct.